Beyond the Hype: How much are businesses actually using AI?
In this article:
How much are businesses using AI?
Why is that?
What needs to happen to make AI more valuable to businesses?
I recently reached out to dozens of advertising, design, and marketing leaders to understand what (if any) AI tools have been effectively built into their workflows. And for most of them -- it was none. And this is in the industries that should have been first-movers in AI.
What does this mean for businesses as a whole?
Well, the spoiler alert is that not only are businesses not adopting AI at the pace that analysts and consultants projected, but AI usage seems to be decreasing from the high point in the last half of February 2024, where 5.8% of US businesses were actively using AI.
Why is this?
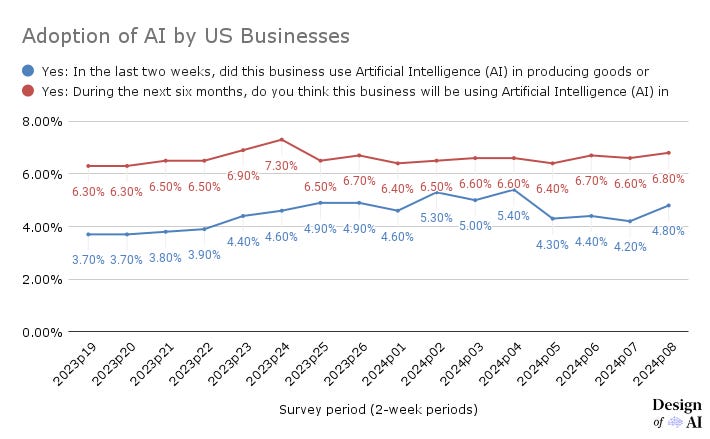
(side note: Many of the reports stating that 40-7x% of employees use AI at work seem to be driven by personal use of GenAI rather than officially sanctioned use. We won’t get into this for today.)
There are several reasons why businesses have been slow to leverage AI:
Data & privacy concerns
Lack of education & training
Prompt engineering has given AI a false barrier to entry
Data quality, availability, and management
Integrating disparate systems
Difficult to identify high-quality, useful AI tools
What can be done to overcome these?
1. Data & privacy concerns
Use AI for non-PII tasks. This can include market research (e.g. IdeaApe) and broader scientific research (e.g. SciSpace).
Use tools like Privacy.AI to securely hide (and annotate) PII before using in more broad-based AI tools. Use video AI tools like Wonder Studio to anonymize biometric data (e.g. customer faces, voices).
Use a private or closed LLM built on an open-sourced model like Llama or through companies like OctoAI, or RunwayML.
2. Lack of education & training
Don't wait for formal training. Consume as much as you can. This means playing with AI tools of all kinds. It means listening to podcasts. It means following newsletters about AI topics.
The ways that AI will shape consumer and business behavior are still unknown, but what we do know is that it will shift the ways in which current roles exist. Get ahead of these changes by learning about AI-related topics outside of your domain; if you're a marketing leader, immerse yourself in product strategy; if you're a product leader, immerse yourself in Data strategy, etc.
Participate in working groups to get hands-on exposure to questions and opportunities that AI can impact, that you wouldn't otherwise participate in.
3. Prompt engineering has given AI a false barrier to entry
Generative AI provides more value the longer you engage with and train it. Leverage it to dig deeper into your ideas, rather than expecting a golden ticket to answers.
Use genAI to critique and push your work further by being your editor, sounding board, and business coach. The biggest criticism of GenAI is that everything becomes average - but this is also why it's particularly adept at knowing when your writing or your argument is too common / cliche.
4. Data quality, availability, and management
It's no secret that the more data an AI is trained on, the more effective it is. However, the common consensus is that you can get results by training a RAG (retrieval augmented generation) instance on as few as 10 documents, as long as they are high quality. This allows the GPT to be trained directly by your private data to inform how it will output responses.
Tools like PDFgear's co-pilot have been trained to act as assistants on single documents, so businesses don't have to be limited to training their own.
Products like Dovetail, Deciphe, QoQo (and many others) allow businesses to use smaller data sets to find patterns and insights into consumer needs and segments.
5. Integrating disparate systems
I personally find this challenge the most frustrating. You either need to let an assistant into everything, or you need to build an ad-hoc toolkit and train multiple resources on your needs and data.
However, this shouldn't be discouraging. The best way to approach this is to map out the workflow of each team in your organization and identify where those team members could use an assistant, a mentor, or a coach. Those will be your quick wins, and where you should focus your efforts to build this "AI toolkit."
6. Difficult to identify high-quality, useful AI tools
Right now this is still a challenge. There are aggregators like There's an AI for that, but that's still limited. Or communities like Hugging Face. But there currently is no "app store" intended to make it easy to browse and make AI tool decisions. In my podcast Design of AI, and in my consulting work with PH1 Research Inc., we're working on a database and resources to make it easier to find high-quality and useful AI tools.
I would love to hear which tools you've found effective and would recommend others. Drop them in the comments.
Brittany Hobbs is a Freelance product insights leader specializing in the adoption of AI at PH1.ca and the co-host of the Designof.AI podcast.